Artificial intelligence has been used in cybersecurity for years, but agencies are increasingly using generative AI for an added layer of protection.
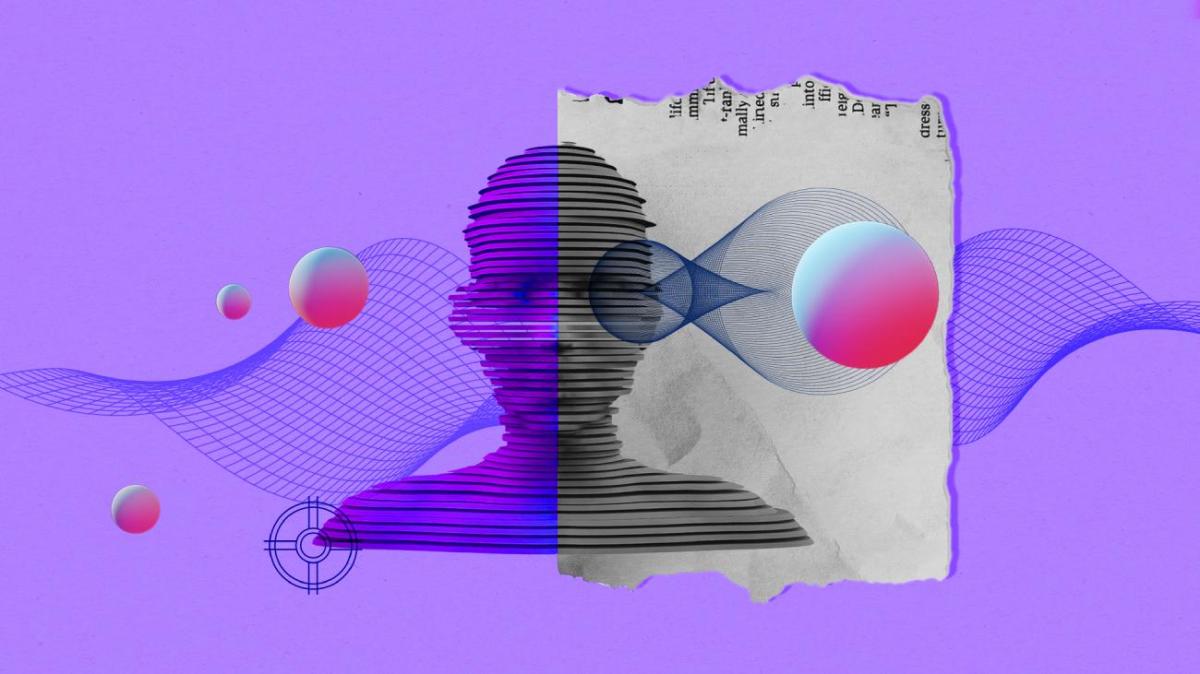
As generative artificial intelligence begins to cement its presence in the operations of state and local governments, other types of AI have already been considered mainstays, particularly in cybersecurity.
Threat detection, incident response and anomaly identification — which all require processing large amounts of data quickly — have used automation and machine learning since the late 1990s. While the boom of generative AI in the fall of 2022 triggered by the public release of OpenAI’s ChatGPT provided opportunities to explore new uses of AI, experts told StateScoop its application in cybersecurity just enhances what automation and machine learning have long been doing.
Cybersecurity officials and analysts said the real power of generative AI in cybersecurity is not in detecting threats, but in synthesizing the data produced by scans for threats and helping cybersecurity experts learn about them contextually. This ability, they said, will help generative AI meet increasingly sophisticated threats, while reducing the need for human intervention.
“From what I’ve seen, as far as cybersecurity tools go, the biggest thing that AI does for us is basically it goes through a lot of data very quickly, and that allows us to contextualize data,” said Andy Hanks, a senior director at the nonprofit Center for Internet Security.
“So, if it’s being used for incident response, or threat hunting, or whatever tool is using it or whatever field in cybersecurity it is being applied to, [generative] AI really changes the game when it comes to processing lots of data very fast and giving you that contextual information about it,” said Hanks, a former chief information security officer for the State of Montana.
Prior to generative AI’s arrival, automation helped cybersecurity experts deploy pattern recognition-based defense systems, also known as expert systems. Expert systems use automation to mimic human expertise, and use data to power task-specific algorithms. While expert systems have been around since the 1970s, their use in cybersecurity in recent decades has been to scan large amounts of data produced by infrastructure scans in search of known threat signatures.
“They’re very rules-based, especially for networks and things of that nature. A good analogy would be like diagnosing things in medicine,” New Jersey CISO Mike Geraghty said. “We get a bunch of expert doctors together, they put in all their findings and all these different roles, and it provides the next doctor using that system with all sorts of rules that he can go by and then make a diagnosis. And that’s the same thing with network security, antivirus or anything like that.”
Expert systems rely on frequent updates to detect new threats. Hanks said he knew of a ransomware attack at an unnamed state several years ago in which threat actors used a known, signature-based virus that should have been detected, but they bypassed the state’s pattern-based system by changing just a few superficial characteristics of its signature. Hanks said it was like the bad actors put a fake mustache on the malware, hoodwinking the detection system.
Joining the stack
Kansas CISO John Godfrey told StateScoop that with how quickly threats evolve now, waiting for the pattern-based automated technology to update and incorporate new threat models into its detection mechanisms is too risky. To overcome this, a standard practice has become to stack automated threat detection tools as filters. Email threat detection, he said, is a good example of how stacked AI technologies are used today in cybersecurity.
“It may be initially spam filter lists, and then it may be Bayesian logic or fuzzy math logic,” Godfrey said of the decision-making frameworks. “And then maybe it’s running it through a couple of different antivirus engines to see if anything still pops out, and then eventually, as it makes its way through the stack, that’s usually how we see a conviction or a result based upon the output.”
Stacks are where generative AI has become helpful. Not only does the contextual information provided — such as a summary of scan findings and recommended defensive actions — help cybersecurity experts better orient themselves and their tools to anticipate future attacks, it also helps with customizing detection systems that can automatically trigger alerts and response actions.
In New Jersey, Geraghty said generative models have been helpful in rewriting threat detection algorithms. While humans can write such computer code, large codebases often become cumbersome — especially when managing several tools in a stack.
“We’ve used code generation from AI,” he said. “So when you think of ChatGPT or any of those things, writing detection rules. Because we think, ‘How do we detect something,’ and you could ask a generative AI tool to be able to write a rule for you in the various types of tools that you’re using.”
‘They’re all using AI’
While the arrival of commercial generative AI has seemed to make the distant, sci-fi reality depicted in movies like The Matrix closer than ever before, AI designed to emulate neural processes and complete complex tasks have been around for decades — even prior to expert systems. Geraghty said the novelty of generative AI has caused some overshadowing of this history and obfuscation of the term “artificial intelligence.”
“I think over the last two years with generative AI, that’s what everybody has come to think of AI being, but there’s probably 70 years prior to gen AI coming on the scene, where all sorts of machine learning models and other AI concepts have been used,” he said.
It comes down to the difference in what the AIs were designed to do, he continued, and that distinction is important for cybersecurity. As implied by its name, generative AI is used to create new content, such as text, image, audio, video or code, in response to specific text prompts. In contrast, automation is designed to streamline complex processes and save time, particularly when it comes to tedious work.
But aside from code generation, generative AI isn’t totally there yet in detecting threats, Geraghty said.
Hanks, the Center for Internet Security director, said one of the first jobs he held in cybersecurity in the 1990s was on an IT server team, where one role was to manually perform the work AI-powered expert systems were originally designed to do: identifying anomalies in large datasets, such as security logs.
“Back then, the very first job that you had on the server team — so whoever the new guy was — it was your job to spend all day going through logs,” Hanks said. “You’d literally sit there for eight hours a day and scroll through logs of all the different servers, looking for problems. And after a while, you got really good at it and you could scroll pretty fast, and your brain would detect breaks in the pattern.”
Eventually, that role was replaced by AI, and over the last 20 years, the use of machine learning has become part and parcel of cybersecurity practices. From basic pattern recognition, identifying known threats and automating routine tasks — cornerstone facets of cybersecurity have all relied on AI.
“Any of those tools and technologies — intrusion detection, antivirus systems — they’re all using some form of AI. It’s just not the generative AI that everybody’s come to know and love lately,” Geraghty said.
An inconsistent model
Even though generative AI’s assistance with contextualizing data seems to be one of its most impactful applications in cybersecurity, other uses are cropping up. One newer use, Godfrey said, is in predictive threat hunting that further limits the need for human intervention.
One method, he said, involves using “honeypots,” trap servers and databases designed to look legitimate. While honeypots have been around for decades, Godfrey said that generative AI is making them better.
“What I’m starting to see is this conversion where we’re starting to see AI that can continuously do threat hunting in the background,” Godfrey said. “And in some cases, start to spin up virtual honeypot in instances that are very contextually based upon the signals are seen from the threat actor and coming in to make it much more enticing for the threat actor to engage with this virtual, fake infrastructure that was created at the time of interaction to divert them away from attacking our core infrastructure.”
Stephen Sims, a fellow at the SANS institute who was one of the first to successfully use ChatGPT to create malware, told StateScoop the practice of stacking generative AI models and deep learning models is becoming more widespread. Such stacking is done similarly to how various automation tools are stacked for email threat detection.
“I’ve also seen companies using various [large language model] agents, each specializing in specific areas of focus, who work together to identify threats,” Sims wrote in an email. “An example could be the teaching of what threat actors look like. This can include running a penetration test or red team exercise against a target environment, where the data is ingested by the various agents in order to be able to determine legitimate traffic versus attack traffic.”
New Jersey has been testing, and recently launched, its own large language model, to which state government employees can ask questions about cybersecurity incident data, Geraghty said. While he said it won’t necessarily generate things like new detection rules, employees can get answers to questions like, “How many ransomware incidents have we seen in the water sector over the past six months?”
Geraghy said the state doesn’t plan to make the tool publicly available, and the model’s inability to return consistent answers indicates how far the generative AI still has to go.
“What we found — and this is common with generative AI — is that if you ask it the same question over and over and over, you get different answers, and that can’t be the case in cybersecurity,” Geraghty said. “A risk assessment that you’re giving to a client has to be spot on. It can’t have superfluous data or hallucinations or anything like that.”
Latest Podcasts
Modernization
Cybersecurity