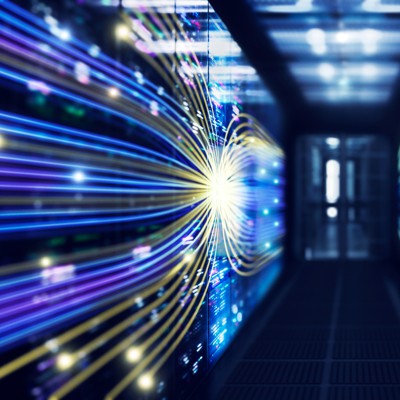
Streamlining the vast troves of existing and incoming data intrinsic to federal operations will be central to agencies’ planned use cases for generative artificial intelligence technologies, federal leadership says, but it is contingent on proper data governance to guard from bias and inaccuracy.
“The primary benefit of using GenAI is the capability of analyzing very vast data sets in government operations and its ability to process and derive insights from those enormous volumes of data,” Chakib Chraibi, chief data scientist at the Department of Commerce’s National Technical Information Service, said at an ATARC panel Thursday.
Internally, agencies are leveraging AI in diverse applications related to this problem. Conrad Bovell, branch chief of cybersecurity advisory and strategy at the Department of Health and Human Services, said that researchers at the National Institute of Health created an AI tool to leverage clinical data to gauge if a given immunotherapy drug would be effective at treating a patient’s cancer.
NIH Senior Data Scientist Nathan Hotaling offered another internal AI application, which would use generative AI software to read unstructured data –– such as notes stored in a PDF document –– and convert it into searchable text and data.
Stephanie Wilson, an agreements officer within the Department of Defense’s Chief Digital and Artificial Intelligence Office, said during the panel that her agency has been using generative AI to do similar work with unstructured data, with the ultimate aim to ease administrative burdens, particularly surrounding contracting paperwork, research and policies.
“I think that kind of impact is really what can help everyone, no matter what their field is, really do their job better, because it used to be reliant on humans to be able to get that contextual information from that unstructured data,” Hotaling said. “And now Gen AI really enables us to use it just like structured information.”
At the National Science Foundation, Chief Information Officer Terry Carpenter said that his agency is looking to deploy a chatbot fueled by large language models, like other agencies, but is focused on specific AI algorithms that use retrieval or predictive analytics for customer experience support.
Federal agencies are also interested in deploying AI in cybersecurity spaces. Bovell cited the double-edged sword AI can bring to such an arena: having cybersecurity teams monitor incoming network data with the help of pattern recognition algorithms, while simultaneously fighting the AI attacks leveraged by cyber criminals and malicious actors.
“AI models can identify patterns indicative of cyber threats such as malware, ransomware or unusual network traffic that might also include data from other existing traditional detection systems,” Bovell said. “Generative AI contributes to more sophisticated analysis and anomaly detection in [security information management] systems as well by learning from historical security data.”
Chraibi said that wrangling some of the outstanding unstructured data in government systems runs parallel to cybersecurity challenges.
“I think the challenges in cybersecurity are kind of similar to what we have in that aspect of data access and management,” he said. “When you talk to a cybersecurity expert, the first thing they complain about is the lack of time, is the lack of bandwidth, that they have. So that’s where I see how generative AI can be leveraged properly, because [generative AI systems] can actually…assist them in doing the tedious work, and then basically elevating the threats where you might expect, [and] can actually tackle them.”
As with any AI application, these federal use cases hinge on how algorithms handle input data and what outputs they generate. While agencies continue to adopt AI capabilities to expedite and facilitate government services, panelists discussed the need to reckon with outstanding security concerns.
Chraibi noted that data poisoning, hallucination and prompt injections can breed harmful or biased AI outputs. Carpenter echoed the lack of standardization in historical government data as an outstanding issue that can negatively impact how an AI algorithm will function.
“The data has been a thorn in our side for decades,” Carpenter said. “We never did finish a job of making the data really tagged appropriately, to understand the data, clean up the data. We need to do some of that.”
He added that, at NSF, the first step to this is cultivating a stronger tech workforce. The agency is supporting curriculum development in prompt engineering to better understand AI and machine learning systems at a holistic level. This involves training everyone in the current workforce on the fundamentals of AI.
“They are different actors involved in different processes with different tools to execute those processes, to really be able to build out these capabilities,” Carpenter said. “IT is not the only party. This is taking even more emphasis off of IT and putting more emphasis back on the mission owner, who understands the data better than IT people to interact with their data on a regular basis and more frequently.”
Chraibi agreed, and reiterated the well-known imperative that a human should be kept in the loop to prevent federal AI solutions from becoming a black box technology.
“[In] the development of GenAI and AI in general, solutions have to be a collaborative effort,” Chraibi said. “The panelists talked about the importance of data quality, so to make sure that that is complete, consistent, unbiased, etc, and that cannot be done just by the data scientists, it has to…get input from the subject matter experts that deal with that type of data.”